
SUPERTUX CHEATS CODE
Follow the instructions on the homework notebook to upload code and data.Select the upload tab then select the.
SUPERTUX CHEATS FREE
When you’re comfortable with the workflow, feel free to use colab notebook (shortened) If you’ve never used colab before, go through colab notebook (tutorial) We provide you with a ipython notebook that can get you started on colab for each homework. You might need a GPU to train your models. Doing so will have negative consequences for your standing in this class and the program. Please do not try to break or hack the grader. print or statements from your code are ignored and not returned.Please do not try to communicate with the outside world. Please do not try to access, read, or write files outside the ones specified in the assignment.Please do not use the exit or sys.exit command, it will likely lead to a crash in the grader.The online grading system will use a slightly modified version of python and the grader: Please contact the course staff before going over this limit, otherwise your submission might be counted as invalid. There is a soft limit of 5 submisisons per assignment. We will use an automated grader through canvas to grade all your submissions. You can compute the IoU and accuracy using the ConfusionMatrix class. You might need to change the class weights of your torch.nn.CrossEntropyLoss, although our master solution did not require this. This metric is harder to cheat, as it computes This is a standard semantic segmentation metric that penalizes largely imbalanced predictions. We additionally measure the Intersection-over-Union evaluation metric. Predicting only track and background gives a $96\%$ accuracy.
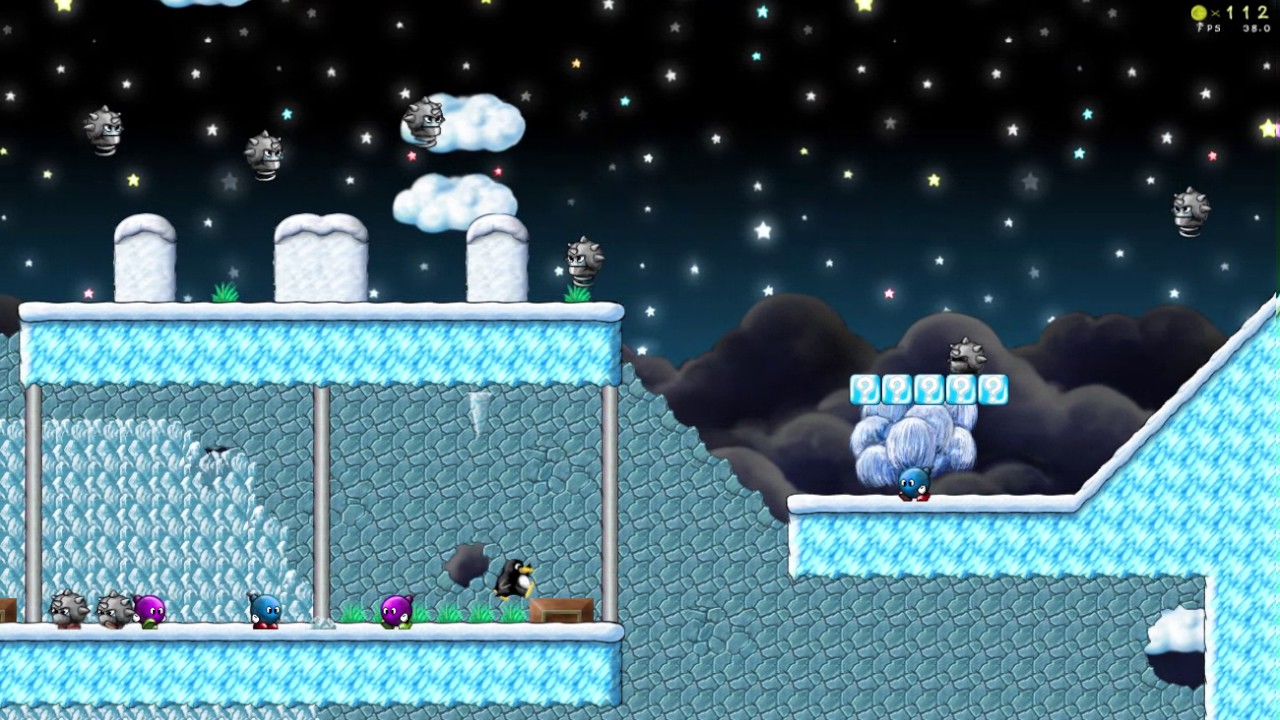
Since the training set has a large class imbalance, it is easy to cheat in a pixel-wise accuracy metric. You will need to use the same bag of tricks as for classification to make the FCN train well. We thus provide you with a smaller subset of useful augmentations that properly work with a pair of image and label in dense_transforms.py. Most standard data augmentation in torchvision do not directly apply to dense labeling tasks. This dataset accepts a data augmentation parameters transform.
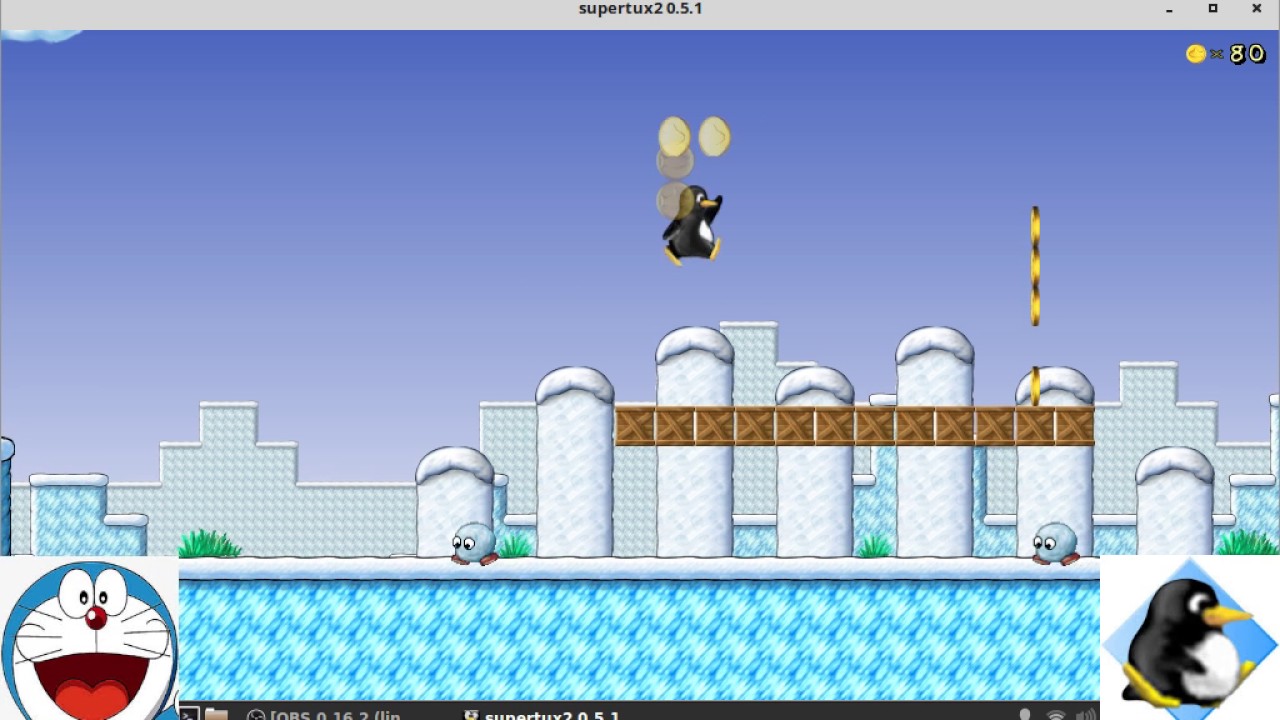
To train your FCN you’ll need to modify your CNN training code a bit.įirst, you need to use the DenseSuperTuxDataset. If you manage to reach this accuracy you’ll get 10 extra credits. We managed to tune our classifier to a $94\%$ accuracy with a training time of 10 GPU minutes. Different levels of supertux have radically different lighting.) Data augmentations (Both geometric and color augmentations are important.

The goal of this first part is to tune the classifier to an accuracy of $90\%$. You may directly copy any code from your solution or the master solution to homework 2. In the first part of this homework, you’ll tune your classifier, or the classifier of the master solution 2 to achieve as high an accuracy as possible. We will check assignments for duplicates. Please, do not directly copy existing code from anywhere other than your previous solutions, or the previous master solution. No collaboration, sharing of solutions, or exchange of models is allowed.
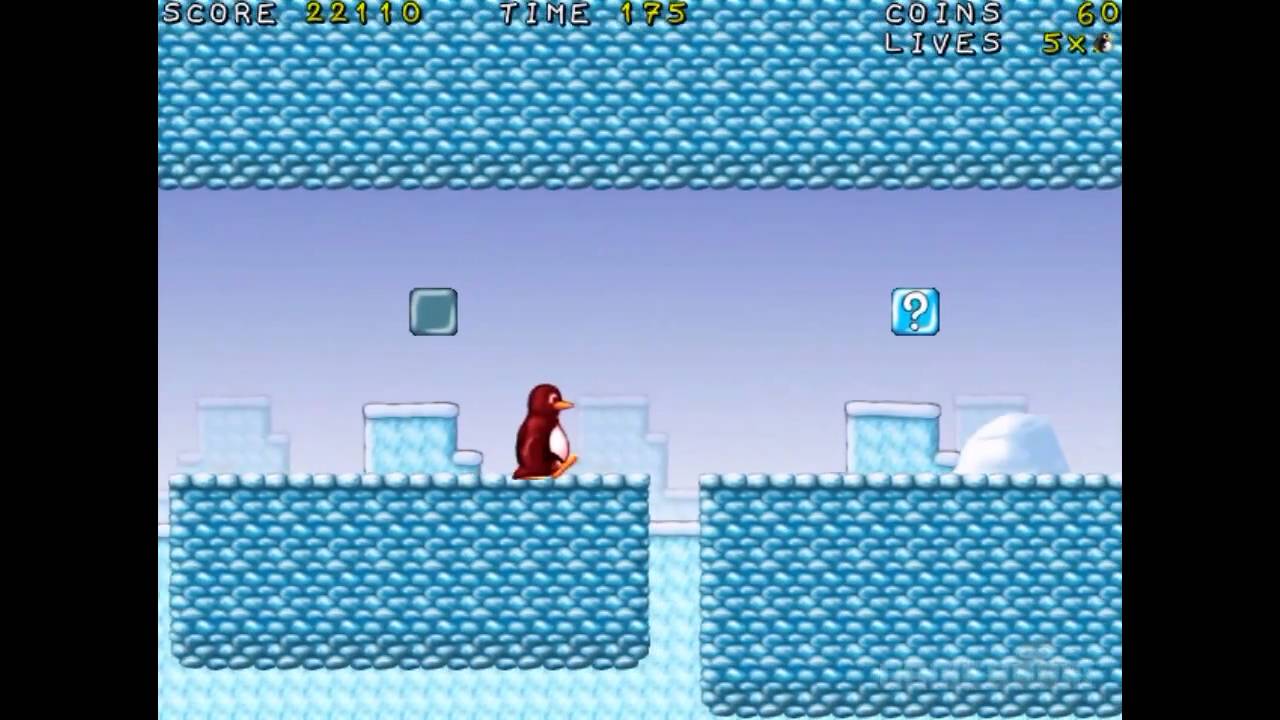
This assignment should be solved individually. We then make this classification network fully convolutional and solve a semantic labeling task (labeling every pixel in the image). In this homework, we will use all the trick at our disposal to tune a classification network on supertux.
